With the rapid development of artificial intelligence technology, explainable AI (xai) has shown great application potential in the financial investment field. xai can help investors better understand the complex mechanisms behind investment decisions, optimize asset allocation strategies, and conduct more robust risk assessments. This article will analyze how xai is applied in portfolio optimization and financial risk assessment based on several industry examples, aiming to provide insights on leveraging xai to empower investment activities.
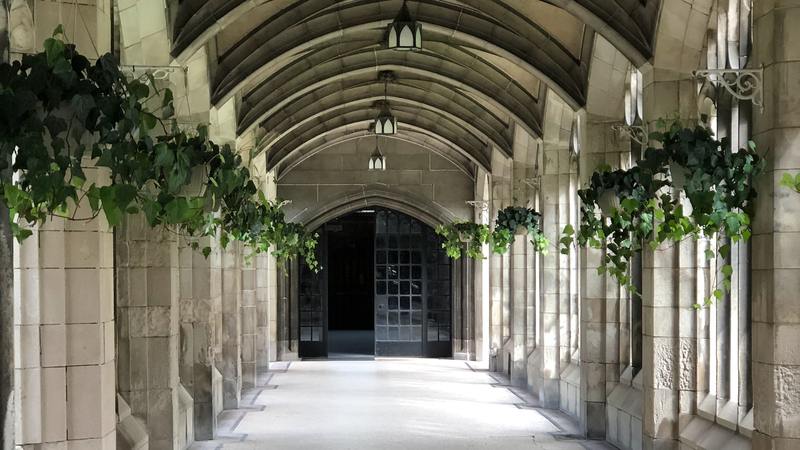
xai reveals driving factors in portfolio optimization
Munich Re, a leading reinsurance company, has developed a xai approach to evaluate the robustness of portfolio diversification resulting from different allocation methods. They found xai models can uncover which market scenarios favor more advanced allocation concepts like Hierarchical Risk Parity (HRP) over traditional methods like Equal Risk Contribution (ERC). This helps identify when and why HRP works better to build more resilient portfolios. Munich Re leveraged SHAP values from XGBoost models to extract feature importance and interactions. The analysis showed max DD and fixed income returns are top drivers of HRP’s outperformance. With GPU acceleration, model training was improved from over 4000 minutes to under 5 minutes, enabling adoption in daily workflows.
xai enables risk assessment automation in insurance
In the insurance industry, xai techniques can be applied to automate and enhance risk assessments for cybersecurity insurance. Startup RiskAssessmentAI built a platform that ingests client security questionnaires and returns precise answers within minutes using AI. This saves significant time and effort compared to manual reviews. The AI models are trained on domain documents to build knowledge and generate checklists and process templates that ensure compliance. TalkingLab is another solution that analyzes call transcripts to surface customer insights and risk factors that can better inform underwriting processes. These examples demonstrate xai’s ability to replicate human judgment in assessing risk and compliance.
xai empowers algorithmic trading strategies
In capital markets, xai can help quants and traders better understand the market conditions and data features driving algorithm performance. For example, Man AHL applied SHAP values to analyze their momentum trading strategy and found that FX carry, equity volatility and credit spreads were top predictors of returns. This transparency helped them refine signal weighting and identify new alpha sources. Singapore’s UOB bank uses xai to analyze customer creditworthiness and fine-tune lending decisions. These use cases highlight how opening the ‘black box’ of AI models enables more contextual and nuanced applications in finance.
xai builds trust and accountability in financial AI
Regulators are increasingly focused on ensuring AI transparency and accountability in finance. The EU proposed the Artificial Intelligence Act with requirements for high-risk AI systems to be transparent, traceable and free of bias. The US Federal Reserve issued guidance for explainability and interpretability in bank AI models. Leading financial institutions recognize explainable AI capabilities are crucial for risk management, compliance and avoiding algorithmic bias. xai will likely emerge as a best practice as its techniques mature. Adopting xai can help investment professionals make more informed decisions and build stakeholder trust in AI.
Explainable AI enables deeper insight into the key drivers behind investment portfolio performance, risk factors and market movements. With compute acceleration, xai workflows can scale to empower everyday applications across organizations. As algorithms grow more complex, xai will become critical for investment professionals to understand, improve and responsibly leverage AI.