With the rise of financial technology and quantitative investing, Python has become an indispensable tool for investment analysis and portfolio optimization. Among the many Python libraries for mathematical optimization, Pulp stands out for its flexibility and ease of use. In this article, we will explore how to leverage Pulp to build investment models and optimize your portfolio allocation. By translating an investment problem into a linear or nonlinear programming model, Pulp allows you to systematically maximize returns under given constraints and risks. Whether you are a beginner looking to enhance your Python skills or a seasoned investor seeking advanced analytical capabilities, mastering Pulp unlocks a whole new approach to making smarter investment decisions.
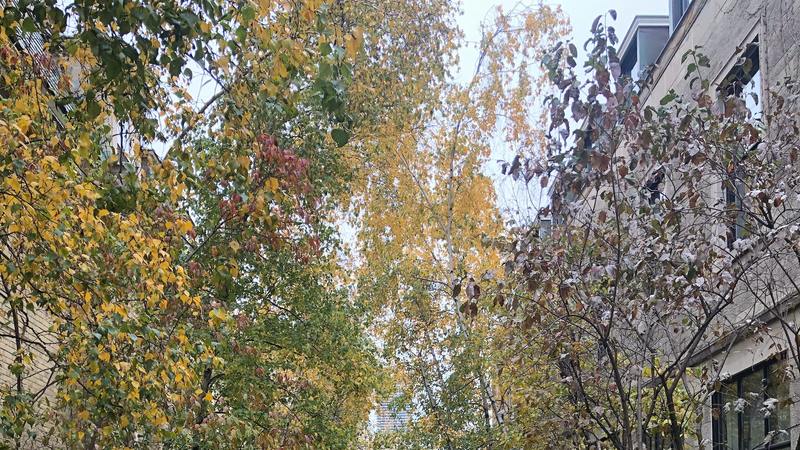
Formulating Investment Problems with Pulp Decision Variables
The first step is to break down your investment dilemma into decision variables that Pulp canoptimize over. For instance, let’s say you want to allocate capital across stocks, bonds and cash to achieve a target return of 6% given your risk appetite. You would define variables like x_stock, x_bond, x_cash to represent the dollar amounts invested in each asset class. Pulp allows you to set variable types like continuous, binary or integer depending on your needs. You can also impose bounds on the variables, like requiring non-negative investments. Defining meaningful decision variables is key to mapping your investment objectives into a mathematical program.
Encoding Investment Constraints and Objectives with Pulp
After defining the decision variables, you need to lay out the constraints and objective for Pulp to optimize. Investment constraints typically limit your overall risk exposure, such as capping the amount invested in any one stock or restricting total portfolio volatility. These constraints are formatted in Pulp using <=, >= and = operators. The objective tells Pulp what to maximize or minimize, like maximizing expected return or minimizing risk. Pulp has convenient methods like LpProblem and LpMaximize to construct your optimization model. The objective and constraints should align with your investment goals, risk tolerance and other requirements. Pulp allows great flexibility here to capture precisely what you want to achieve in your portfolio.
Obtaining the Optimal Portfolio Mix with Pulp Solvers
After coding up the variables, constraints and objective, you can call Pulp’s solve() method to obtain the optimal investment portfolio. Pulp supports various linear and nonlinear solvers like GLPK, COIN-OR and CPLEX to crunch the numbers. The solved values of your decision variables indicate how much to allocate to each asset class. You can also access useful results like the maximized objective value, i.e. expected return. The optimization results quantify the best portfolio composition that satisfies your real-world requirements. As you refine the model, Pulp will keep uncovering improved solutions tailored to your needs.
Backtesting and Iterating Pulp Investment Models
To validate your Pulp portfolio model, it helps to backtest it against historical data. You can compare your model’s simulated returns to actual returns over a past period. This reveals how your model would have performed and allows tweaking it to improve predictions. You may adjust the objective function, refine constraints or add new factors like transaction costs. The flexibility of Pulp enables iterating models quickly until you arrive at robust solutions. Backtesting facilitates turning a sound investment theory into practice by ensuring good performance on real data.
Pulp is an invaluable addition to any investor’s Python toolkit. It facilitates optimizing portfolios programmatically to systematically enhance returns while managing risks. With Pulp models, you gain an advantage over qualitative investment approaches prone to behavioral biases. Hands-on practice with Pulp allows developing customized and sophisticated models not possible with off-the-shelf tools. Pulp offers a proven way to boost returns for both retail and institutional investors.