Rules based investing refers to investment strategies that adhere to predefined rules and models for selecting assets or making trades. It relies on quantitative analysis and systematic rules instead of subjective decision making. This approach has become increasingly popular with the rise of algorithmic trading, robo-advisors, and smart beta ETFs. In this article, we will explore some key concepts and practical examples of rules based investing strategies across different asset classes like stocks, bonds, real estate etc. Proper implementation requires meticulous design of robust rules, predictive signals, risk controls and portfolio construction. When executed well, rules based strategies can provide stable long term performance less prone to behavioral biases.
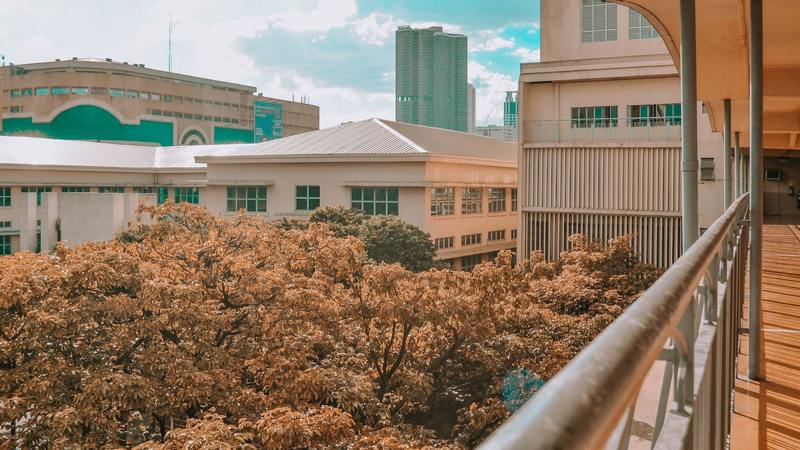
Trend following rules exploit persistent directional movement
One of the most common rules based technique is trend following, which simply invests in the direction of a prevailing price trend. For example, a moving average crossover system will generate buy and sell signals based on a fast moving average crossing above/below a slower moving average. Commodity trading advisors (CTAs) and managed futures funds often use such momentum rules on diverse markets. Trend following works well when markets exhibit persistence in one direction but can underperform in choppy or reversing markets.
Mean reversion betting counters overextensions
In contrast to trend following, mean reversion strategies bet that prices will revert back towards historical averages after sharp deviations. For instance, a price to book ratio (P/B) model would take long positions in extremely undervalued stocks and short overvalued ones based on comparison to average valuation. Fixed income relative value trades that capitalize on temporary mispricings are an example. The key is identifying when distortions truly are excessive rather than new normal.
Combining complementary rules improves results
Since individual rules have pros and cons, sophisticated funds combine complementary rules to improve results. For example, adding mean reversion elements can make trend following more robust. Machine learning techniques can also optimize rule combination. But the principles of dimensionality reduction applies i.e. finding the smallest set of predictive signals is best to prevent overfitting.
Risk control rules essential for resilience
No rules based system can be foolproof in catching every market twist so strict risk control rules are essential. Stop loss exits, position sizing, volatility scaling, diversification constraints all play a role in limiting drawdowns when the Unexpected happens. Systematic rebalancing is also needed to prevent realized risks from deviating greatly from planned ones.
Portfolio construction ties together objectives
Bringing together the various rules and positions requires the art of portfolio construction to create a cohesive rules based strategy matching investor objectives, risk appetite and constraints. This includes combining rules with complementary exposures, offsetting risks, aligning frequencies, assigning appropriate capital etc. Fine tuning these portfolios requires experience along with backtesting and stress testing their performance.
In summary, rules based investing encompasses a methodology to systematically apply quantitative rules over discretionary choices. Proper implementations require robust design of predictive signals, prudent risk control guardrails, and thoughtful portfolio construction. When executed well, rules based strategies can generate stable returns less prone to behavioral biases but still carry risks of model failure. Trend following and mean reversion are two common examples but combinations with machine learning and risk overlay hold promise.