Quantitative equity investing, also known as algorithmic or systematic trading, has become increasingly popular among hedge funds and institutional investors. It utilizes mathematical models and statistical algorithms to make investment decisions, allowing for quicker reactions to market opportunities and more disciplined risk management. In this comprehensive guide, we will explore the key concepts, strategies, and considerations for building a successful quantitative equity investment process.
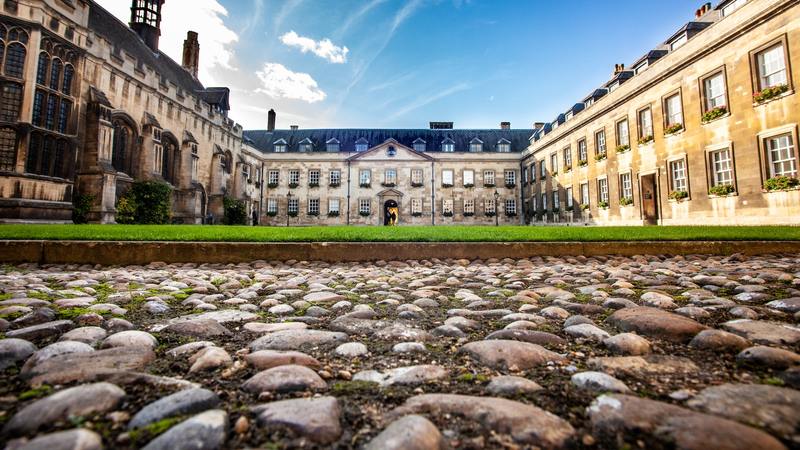
Common quantitative equity strategies
Some of the most common quantitative equity strategies include:
– Statistical arbitrage – Identifying short-term mispricings between correlated securities and profiting from the convergence of prices. This could involve pairs trading between two stocks or dispersion trading across a basket of securities.
– Trend following – Using moving averages or other indicators to determine the overall market trend and taking directional long or short positions accordingly. Risk is controlled by cutting losses when the trend changes.
– Mean reversion – Contrarian trading around a stock’s historical average valuation. Securities that have diverged too far from typical levels are expected to revert back over time.
– Factor models – Combining factors like value, momentum, quality, volatility that have demonstrated historical efficacy in predicting returns. Multifactor models help diversify and enhance performance.
– Machine learning – Applying AI/ML algorithms to uncover non-linear relationships and hidden patterns in market data that are difficult for traditional quant models to detect.
Essential components of a quantitative equity system
Building a robust quantitative equity investment process requires expertise across multiple disciplines:
– Financial engineering – Constructing mathematical models, statistical algorithms, and portfolio optimization techniques.
– Data science – Collecting, cleaning, and analyzing large amounts of structured and unstructured data.
– Computer science – Developing fast, scalable, and reliable software infrastructure for research, trading, and operations.
– Portfolio management – Combining multiple alpha signals, optimizing tradeoffs between risk and return, and minimizing transaction costs.
– Trading infrastructure – Low-latency connections to markets, smart order routing, and position sizing based on liquidity.
– Risk management – Guardrails for position sizing, diversification, drawdowns, and tail risks.
– Compliance – Navigation of regulatory requirements across multiple jurisdictions.
Common challenges in quantitative equity investing
While quantitative strategies offer many advantages, they also come with unique challenges that need to be addressed:
– Overfitting – Developing excessively complex models that degrade out-of-sample. Robust cross-validation techniques are essential.
– Data mining – Testing multiple hypotheses on the same limited dataset leads to false discoveries. Need to separate research and live trading.
– Crowding – Similar quantitative signals across different funds leading to overcrowded trades. Uncorrelated unique signals are key.
– Model decay – Strategies stop working over time as other investors start exploiting the same signals. Requires constant innovation and updating.
– Unexpected tail risks – Quant models optimized for normal environments can blow up during market dislocations outside historical regimes.
Best practices for running a quantitative equity business
Successfully operating a quantitative equity investing firm over the long run requires following certain best practices:
– Specialized team – Hire exceptional data scientists, technologists, researchers, portfolio managers, and traders with expertise in systematic investing.
– Research focus – Devote significant resources to research and development of new strategies.
– Disciplined execution – Follow models and rules-based approach without emotions or discretionary overrides.
– Risk control – Robust risk management integrated throughout the process, not just tacked on.
– Technology – Cutting-edge proprietary infrastructure to enable rapid research iteration and scalable implementation.
– Operational diligence – Meticulous attention to details and processes across the entire investment lifecycle.
– Continual improvement – Relentless drive to make strategies more efficient and effective over time.
– Thoughtful growth – Grow carefully and remain nimble. Prioritize quality over asset gathering.
Quantitative equity investing provides mathematically-driven rules-based approaches to find and exploit edges in the market. With the right expertise, rigorous research, disciplined execution, and cutting-edge technology, alpha can be generated systematically at scale. However, quant models have inherent risks requiring robust risk management. Long term success requires constant innovation, learning, and improvement.