Quantitative bond investing has grown in popularity in recent years as a way to systematically manage bond portfolios. It relies on mathematical and statistical models to evaluate bonds, construct optimal portfolios, and implement dynamic trading strategies. By leveraging algorithms, big data, and computing power, quantitative bond funds aim to maximize risk-adjusted returns. This approach stands in contrast to traditional fundamental bond analysis and allows for more precision in managing factors like duration, credit, curve, and liquidity risk. In this article, we provide an overview of popular techniques in quantitative bond investing such as risk parity strategies, statistical arbitrage, and algorithmic trading systems. We also discuss challenges such as leverage constraints, model risk, and regulatory shifts that quants must navigate.
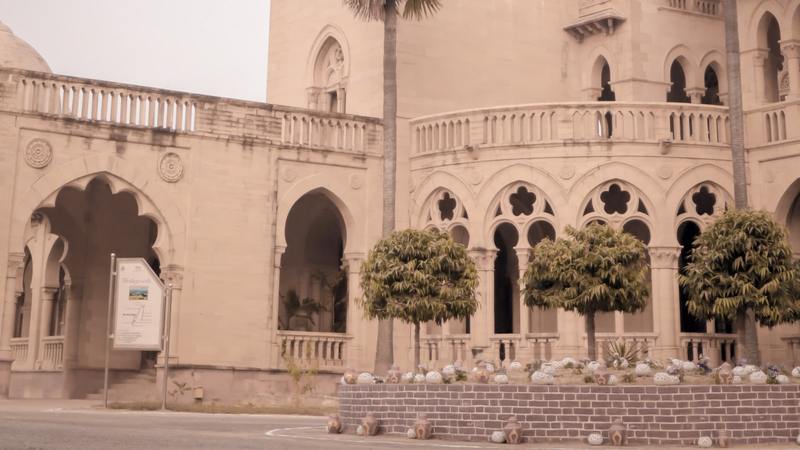
Risk parity strategies for equal weighting bond risks
One main technique in quantitative bond investing is risk parity. As opposed to traditional bond funds that are overweight equities, risk parity seeks to equalize risk across asset classes by leveraging up low-risk bonds. This means allocating more capital to traditionally defensive fixed income so that portfolio losses are not dominated by equities in a market downturn. Bridgewater’s All Weather strategy exemplifies this approach. By identifying various economic regimes, the portfolio can be built to withstand different environments. Cliff Asness also showed how applying leverage to lower-risk assets like Treasuries can optimize returns for a given risk budget. Challenges for risk parity include leverage constraints that hamper expected returns and model uncertainty in forecasting macro regimes.
Statistical arbitrage strategies profiting from bond mispricings
Another quantitative approach sees bonds and fixed income assets as being prone to temporary mispricing that can identified and profited from. For example, on-the-run Treasuries frequently trade special in terms of liquidity premiums due to intense institutional demand. As a new issuance becomes the benchmark on-the-run, the previous one becomes rich in yield relative to modeled fair value. A common statistical arbitrage strategy would be to short the expensive bond and buy the cheap one, betting on convergence. More elaborate multi-asset stat arb strategies might involve interest rate derivatives, swaptions, or yield curve trades alongside cash bonds. Challenges involve managing risks since convergence may take time and fundamentals can shift. There is model risk as well in terms of pricing anomalies being ephemeral.
Algorithmic and high-frequency trading systems
Quantitative bond investing also consists of automated algorithmic trading systems that scan markets to implement favorable trades, as well as high-frequency strategies that exploit microstructure and latency arbitrage opportunities. These can involve simple tactics like VWAP trading or more advanced machine learning algorithms trained on price data to predict optimal entry and exit points. Some fixed income algorithms are liquidity providers serving client order flow on electronic platforms in exchange for spread capture. Others are predatory algorithms seeking to detect institutional order flow and trade ahead. As electronic trading grows across corporate bonds and emerging markets, the scope for algorithms expands. Challenges center around adapting to evolving microstructure, competition from rival algorithms, and technological failures that can lead to unintended market impacts or compliance breaches.
In summary, quantitative bond investing encompasses various strategies from risk parity asset allocation to statistical arbitrage strategies across asset classes to high-frequency trading algorithms. By applying advanced statistical and computational techniques, quantitative bond funds seek risk-adjusted return optimization. However, successful deployment requires managing model risk, constraints around leverage and liquidity, regulatory shifts, and technology dependencies.