Making sound investment decisions is crucial yet challenging, as it involves multiple complex criteria and inherent uncertainties. Pythagorean fuzzy set theory, an extension of intuitionistic fuzzy sets, provides a useful mathematical framework for modeling imprecise and ambiguous information in investment decision making. In this article, we will explore how Pythagorean fuzzy numbers can help address limitations of traditional methods and enable more informed decisions when investing.
The key advantage of using Pythagorean fuzzy numbers lies in their ability to better capture the vagueness and imprecision of decision data. By using both membership and non-membership functions whose sum of squares is less than or equal to 1, Pythagorean fuzzy sets can describe complex interactions between criteria more accurately than traditional fuzzy sets. This provides more nuanced modeling of uncertainty and allows for finer-grained analysis when comparing investment options.
Additionally, Pythagorean fuzzy aggregation operators, such as weighted averaging, geometric, and Einstein operators, offer effective ways to synthesize multiple criteria into an overall assessment. The induced heavy aggregation operators also consider interrelationships among criteria, leading to more robust evaluation. By applying Pythagorean fuzzy decision making methodology, investors can systematically assess alternatives and make optimal choices aligned with their goals and risk preferences.
In the following sections, we will showcase Pythagorean fuzzy decision models for stock selection, venture capital decisions, supplier selection in portfolio management, and more. The case studies demonstrate how Pythagoran fuzzy sets enable more informed, mathematically grounded decisions, better reflecting the complex realities of investing.
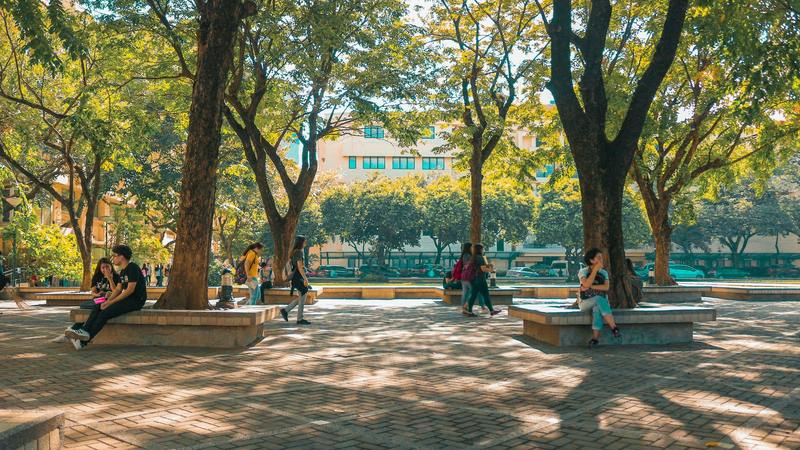
Using Pythagorean fuzzy TOPSIS method for stock selection
Selecting stocks to invest in involves evaluating companies across various criteria like financial ratios, management quality, growth potential, etc. It is an inherently complex decision with significant uncertainty and risks. The Pythagorean fuzzy TOPSIS (Technique for Order of Preference by Similarity to Ideal Solution) approach allows a more rigorous stock selection process:
Step 1: Construct a Pythagorean fuzzy decision matrix. Financial experts assess each stock against criteria like PE ratio, ROE, market share etc. using Pythagorean fuzzy numbers that capture the imprecise evaluations.
Step 2: Determine weights of each criterion based on their relative importance. Geometric aggregationoperators can derive weights from the Pythagorean fuzzy decision matrix itself.
Step 3: Identify positive and negative ideal solutions. The best (positive) and worst (negative) performing stocks across each criteria are theoretical benchmarks.
Step 4: Calculate separation measures of each stock from the ideal solutions. Pythagorean fuzzy weighted Euclidean distance method helps quantify gaps.
Step 5: Evaluate relative closeness coefficients of each stock to the positive ideal solution. The coefficients determine rankings of stocks, with higher values indicating better overall performance.
By leveraging Pythagorean membership, non-membership and hesitation degrees, this method provides greater modeling fidelity. The TOPSIS flow also offers a systematic quantitative approach for stock prioritization and selection versus intuitive judgment.
Applying Pythagorean fuzzy AHP in venture capital investment decisions
Venture capital firms need to meticulously evaluate startups on criteria like innovation, growth potential and team strengths before investing in high-risk, high-reward deals. Pythagorean fuzzy analytical hierarchy process (AHP) enables sound judgment for these complex decisions:
Step 1: Break down the decision problem into a hierarchy with the goal at the top. Level 1 has key criteria for evaluating startups – technology innovativeness, market potential, management capability etc.
Step 2: Construct Pythagorean fuzzy pairwise comparison matrices to derive weights of the criteria. Domain experts compare criteria using Pythagorean fuzzy numbers to capture ambiguity.
Step 3: Check consistency of judgments. The Pythagorean fuzzy AHP methodology ensures logical, consistent inputs.
Step 4: Evaluate each startup against the criteria using Pythagorean fuzzy ratings. Aggregate the ratings into overall scores using weighted arithmetic averaging or Einstein operations.
Step 5: Rank the startups and select the most promising one(s) based on the overall scores. Sensitivity analysis provides insights on decision stability.
The Pythagorean fuzzy AHP approach enables systematic, mathematically rigorous modeling of the complex qualitative factors VC investors consider. By capturing greater nuance and inconsistencies, it leads to better informed investment decisions.
Using Pythagorean fuzzy Aggregation in portfolio management
Portfolio managers need to diligently evaluate and select suppliers, such as brokers, financial advisors, data providers etc. based on criteria like reputation, services offered, fee structures and customer satisfaction ratings. Pythagorean fuzzy aggregation operators help handle such multi-criteria decisions involving uncertain, subjective assessments:
Step 1: Construct the Pythagorean fuzzy decision matrix with supplier assessments against each criterion provided by experts using Pythagorean membership, non-membership and hesitation degrees.
Step 2: Determine objective weights of the criteria based on their relative importance to the supply chain.
Step 3: Aggregate the matrix information using Pythagorean fuzzy weighted averaging or geometric operator to derive overall performance scores of suppliers. Einstein operations can also be used.
Step 4: Rank the suppliers based on the scores and select optimal partners.
By considering interactions between criteria and leveraging both membership and non-membership functions, the Pythagorean fuzzy aggregation approach leads to more robust supplier selections in portfolio management. The method is also relatively simple and fast compared to more complex optimization models.
In summary, Pythagorean fuzzy set theory provides a useful framework to address the challenges of imprecision, uncertainty and subjectivity in investment decision making. Pythagorean fuzzy numbers, aggregation operators and decision models lead to more rigorous analysis by providing greater fidelity in capturing ambiguities and complex interactions. The case studies on stock selection, venture capital decisions and portfolio management demonstrate the methodology’s effectiveness in enabling more informed, mathematically optimal investments aligned with sound judgment.