With the development of big data technology, data analysis has become an indispensable tool for private real estate investment activities. Effective use of data can help investors better understand market conditions, find potential opportunities, evaluate projects, optimize portfolios and make informed decisions. This article will focus on private real estate investment data analysis methods and approaches that lead to optimal decisions. Data sources include public records, proprietary databases, field surveys and more. Analytical techniques like regression analysis, scenario analysis and simulation can reveal insights for acquisition, financing, operation and disposition. By leveraging comprehensive datasets and analytical models, investors can identify sound investment opportunities, assess risk-return trade-offs, enhance property management, and maximize investment returns. With plenty of data and rigorous analysis, investors can make the right choices at each stage of the real estate investment cycle.
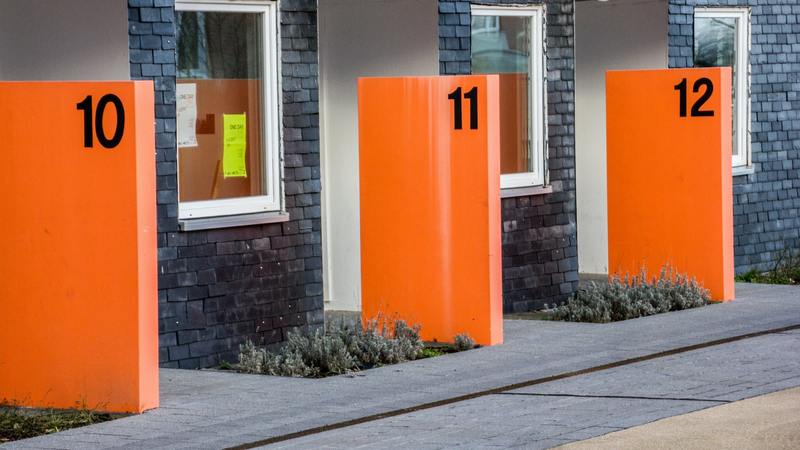
use comprehensive data sources to understand private real estate investment market
A wide range of data sources should be utilized to fully understand the private real estate investment market. Public records like zoning maps and transaction records provide information on comparable properties. Proprietary databases from data vendors track property details, rents, vacancies, tenants etc. Field surveys by research teams give on-the-ground insights. News and industry reports analyse trends and forecasts. Demographic and employment data reveal population, income, job changes that impact real estate demand. Macroeconomic factors like GDP, interest rates and policy changes indicate investment risks. Environmental databases assess sustainability. Crowdsourced data uncovers consumer preferences. The more comprehensive the data, the better investors can gauge market conditions and find potential opportunities.
use regression analysis and forecasting for private real estate investment valuation
Regression analysis is an important technique for private real estate valuation and forecasting. By analysing historical data on property prices, rents, vacancies and other factors, regression models can identify drivers of real estate value and their impact magnitudes. Key independent variables may include property type, size, age, location, income, interest rates. Regression equations can predict property prices and rental income for discounted cash flow analysis. Forecasting models can also project future trends based on macroeconomic scenarios. Different assumptions can be simulated to evaluate investment risks. With large real estate data samples, multi-factor regression models allow in-depth analysis of valuation and forecasting elements for each property type and local market.
leverage data-driven analysis for optimal private real estate investment portfolio strategy
Data analytics enable private real estate investors to pursue optimal portfolio strategies that maximize risk-adjusted returns. Based on individual project underwriting, aggregation analytics assess a portfolio’s overall risk profile, diversification, cash flows, return expectations vis-à-vis targets. Asset allocation modelling provides data-driven insights on balancing different property types, geographic locations, risk-return profiles, development stages. Stress testing simulates portfolio performance under adverse scenarios. Ongoing monitoring analytics track asset concentrations, cash balances, leverage metrics. As portfolio conditions evolve, analytics inform strategic moves to rebalance assets or tap refinancing/disposal options. Big data allows analysis of interlinkages between macro factors, local market dynamics and property fundamentals to arrive at portfolios resilient across market cycles.
use data analysis to enhance private real estate investment operations and management
In-depth data analysis also improves private real estate operations and property management. Data-driven approaches can optimize leasing strategies by identifying most lucrative tenant mixes, rental rates, lease structures, and concessions based on granular demand insights. Operating cost analytics spots redundancies and guides expense optimization. Data identifies preventative maintenance needs before problems arise and avoids emergency costs. Usage data patterns allow tailoring amenities to tenant preferences. Data-enabled smart building technologies cut waste and costs. By leveraging analytics, investors can thus enhance property competitiveness, tenant experience, cost efficiency and ultimately, investment returns.
implement data-driven decision-making across the private real estate investment lifecycle
In summary, data analysis should drive decision-making throughout the private real estate investment process. In acquisition, it enables prudent project selection and pricing based on market analytics. In underwriting, it allows proper risk-return calibration through scenario testing. For financing, it informs optimal debt choices by revealing relationships between leverage, interest rates and returns. In operations, it uncovers cost savings and revenue opportunities. In disposition, it identifies optimal timing to meet return targets and rebalance portfolios. With each decision based on robust data insights rather than gut feel, investors can build resilient portfolios. A highly analytical approach is key to long-term outperformance in private real estate investing.
In conclusion, private real estate investors should tap into comprehensive data sources and analytical techniques to make informed decisions at every stage. By leveraging extensive data on markets, properties and external factors along with rigorous modelling, they can identify sound investments, forecast value, optimize portfolios, enhance operations and maximize risk-adjusted returns.