Parametric investment has become an increasingly popular technique for portfolio construction and optimization in recent years. By using statistical and quantitative methods, investors can systematically select and weight assets to achieve target risk-return profiles. In this article, we will explore the methodology behind parametric investment strategies, including key concepts like mean-variance optimization, factor models, and leverage constraints. Proper application of parametric approaches can lead to well-diversified, risk-managed portfolios tailored to an investor’s goals. However, there are also downsides like model risk that need to be considered. Overall, parametric investment offers many advantages but requires rigorous quantitative skills to implement successfully.
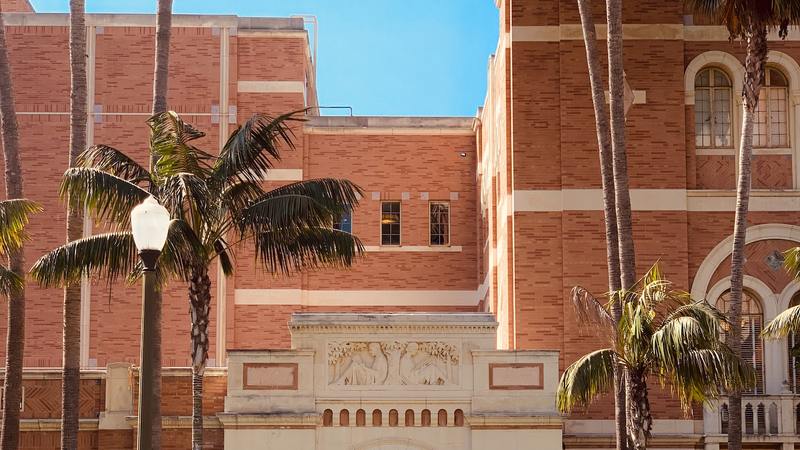
Mean-variance optimization forms basis of parametric investment strategies
Modern portfolio theory pioneered by Harry Markowitz introduced the idea of combining assets in a portfolio to optimize the tradeoff between risk and return. His Nobel prize winning work on mean-variance optimization provides a mathematical framework for investors to construct optimal portfolios given expected returns, volatilities and correlations between assets. By minimizing portfolio variance for a target level of return, efficient frontiers defining risk-return profiles can be generated. Parametric investment strategies leverage these mean-variance optimization techniques to systematically build portfolios. However, some limitations exist in practice due to difficulty of accurately estimating the large number of parameters required.
Factor models improve diversification and risk management
Rather than forecast expected returns individually for each asset, parametric investment strategies often use factor models that identify common drivers behind asset returns. For example, the Fama-French three factor model incorporates market, size and value factors. By focusing on a small number of key factors, the inputs and complexity for mean-variance optimization are greatly reduced. This allows for improved diversification and risk management during portfolio construction. However, model risk exists if the chosen factors do not sufficiently explain returns.
Leverage constraints help avoid excessive risk taking
Unconstrained optimization models can sometimes result in large negative portfolio weights, essentially representing high leverage. However, highly leveraged portfolios can suffer dramatic losses if asset prices decline. Parametric investment strategies should incorporate leverage limits to avoid excessive risk taking. This can be achieved by directly constraining the sum of negative weights or by penalizing leverage in the objective function. Though leverage caps reduce possible returns, they help ensure stable risk profiles aligned with an investor’s risk tolerance.
Big data and machine learning will transform parametric investment
The growing availability of big data across markets combined with advances in machine learning are opening new possibilities for parametric investment strategies. Instead of relying on simplified factor models, algorithms can now identify complex patterns and dynamics driving asset returns based on large datasets. Portfolio optimization can then be enhanced using robust machine learning techniques while adapting to changing market conditions. However, overfitting and interpretation remain key challenges. Overall, integrating big data and AI into parametric investment strategies offers avenues for better portfolio construction but requires careful statistical validation.
Parametric investment methodologies leveraging optimization, factor models and constraints allow for systematic and rules-based portfolio construction tailored to investor goals. However, quantitative rigor is essential to implement these strategies successfully and achieve the promised benefits versus traditional active management. Continued adoption of big data and machine learning provides opportunities to further enhance parametric approaches in the future.