Recently, machine learning has shown great potential in optimizing various aspects of real estate investing. By leveraging large datasets and advanced algorithms, machine learning models can help real estate investors identify lucrative investment opportunities, analyze property valuation, predict market trend and risk, automate due diligence, and enhance overall portfolio performance. Though still an emerging application, early adopters of machine learning have reported improved investment returns and operational efficiency. This article will provide a comprehensive review of the state-of-the-art machine learning techniques for real estate investment, and discuss their benefits, limitations and future outlook of this promising technology.
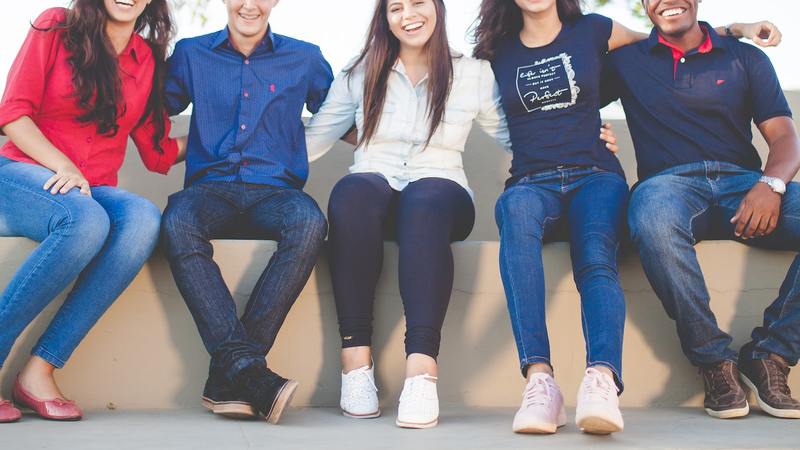
Automated property valuation using machine learning leads to more accurate pricing
One major application of machine learning in real estate investing is automated valuation modeling (AVM). Traditional AVMs rely on simple regression analysis of limited property attributes like size, age, neighborhood, etc. Advanced machine learning techniques such as random forest, gradient boosting machine or neural networks can assimilate more data like prior sales history, tax records, geographic & demographic variables, and even image recognition of property photos to construct robust valuation models with higher accuracy. For example, a startup named HouseCanary applied deep neural networks to value residential properties in the US with 95% accuracy. More accurate property pricing helps investors identify undervalued properties with strong rental income or resale potential.
Machine learning-powered property search engine improves deal sourcing
Sourcing profitable investment properties is critical yet challenging in real estate investing. Traditional methods like brokers or MLS listing are inefficient. With web scraping and natural language processing techniques, machine learning models can scrape online property listings from multiple sources, extract key attributes via text analysis, categorize and filter them to match each investor’s unique investing criteria (e.g. price range, property type, rental yield, rehab costs etc.). This automated deal-sourcing pipeline significantly improves the quantity and quality of deals individual investors can discover, which lays the groundwork for successful property investing.
Predictive analytics on real estate data detects local price trend and downturn risks
Real estate exhibits strong local characteristics – property values depend on micro-level demand-supply dynamics within each neighborhood. Machine learning models can combine and analyze granular data like rental listings, home sales, online search traffic, household formation and employment statistics to uncover hyper-local trend and predict future price changes at the zip code or even property level. Such predictive analytics empowers investors to identify emerging neighborhoods with strong upside potential early on. Likewise, machine learning algorithms can also detect early signals of impending price correction or downturn risks in a local market using similar data analytics, allowing investors to adjust their strategy accordingly.
Automated due diligence reduces rehab costs and risks for fix-and-flip projects
Fix-and-flip relies on accurate property inspection and cost estimation to ensure profitable business case after rehab. Traditionally this requires extensive manual work and expert knowledge. With computer vision and deep learning techniques, investors can automate analysis of property photos to detect required repairs, estimate repair costs, and pinpoint potential deal-breaking issues early, reducing costly surprises down the road. Some startups also use drone videos and 3D home scans to construct comprehensive digital twin of target property, allowing remote inspection and rehab planning. This reduces manual work as well as risks of unforeseen rehab costs and delays.
Machine learning is transforming real estate investment landscape and generating immense values to investors through superior analytics and automation. However current applications focus more on operational enhancement rather than high-level strategy optimization. With continued advancement in artificial intelligence, machine learning has the potential to become an indispensable assistant that provides data-driven insights on dynamic investment strategies, portfolio optimization and risk management for real estate investors.