With the rapid development of artificial intelligence and big data technology, machine learning has become an increasingly important tool for investment analysis and decision making. As one of the largest investment banks, JP Morgan published an influential 280-page guide on using machine learning in finance. In addition, there are many insightful notes and summaries written by professionals and students to explain key machine learning concepts and their applications in investing. By studying these materials, investors can gain valuable insights on leveraging machine learning algorithms to process alternative data, identify patterns and make more informed investment decisions.
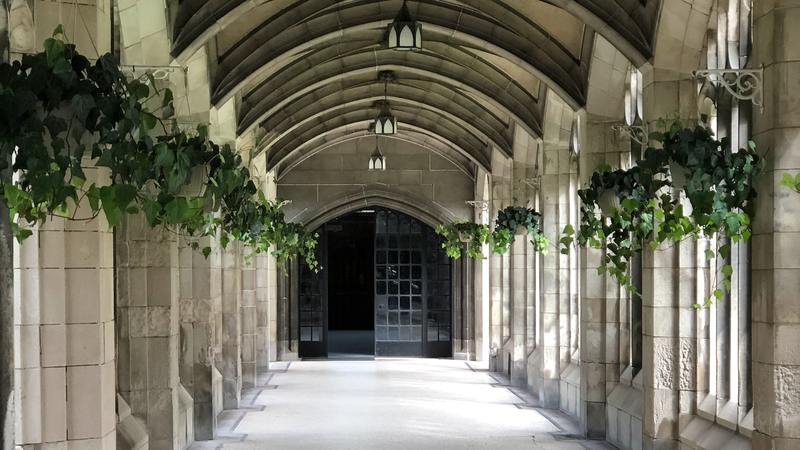
Supervised learning helps predict investment variables like returns
The JP Morgan guide points out supervised learning is useful for establishing relationships between datasets, like using historical data to predict returns. Regression methods model continuous outputs like stock price changes, while classification models categorical outputs like market turning points. Overall, supervised learning excels at finding predictive signals in data.
Unsupervised learning reveals hidden relationships and main drivers
Unsupervised learning methods like clustering and factor analysis help investors identify hidden patterns in data without predefined outputs. For example, clustering can reveal different market regimes, and factor analysis can uncover the main drivers behind yield curve movements. This allows discovering new insights from big datasets.
Deep learning systems mimic human intelligence for sophisticated tasks
Deep learning uses neural networks loosely inspired by the human brain to undertake sophisticated tasks that are easy for humans but hard to explicitly program, like analyzing news and earnings reports. According to JP Morgan, deep learning is well-suited for making probability estimates, like predicting market corrections.
Reinforcement learning optimizes trading strategies through trial and error
Reinforcement learning models can simulate different trading strategies to dynamically find the most profitable actions over time through trial and error. Without knowing the optimal decision at each point, reinforcement learning allows ‘practice makes perfect’ to incrementally improve strategies.
Key is marrying data science skills with market knowledge
The JP Morgan guide emphasizes effective data analysis requires both strong data science abilities and solid grasp of market fundamentals. Machine learning models are tools for digesting data, not oracles. Domain knowledge remains essential for strategy and interpretation.
In summary, these machine learning notes highlight key techniques like supervised learning, unsupervised learning, deep learning and reinforcement learning all have valuable yet distinct applications for investment analysis and decision making. But fully capitalizing on them requires combining mathematical rigor with intuitive market understanding.