With the availability of big data and advancement in computing power, machine learning has become an important tool for quantitative investing and factor investing. Machine learning can help detect hidden patterns and dynamics beyond the normal factor return anomalies. It also provides the flexibility to adapt models to evolving market conditions through retraining. This article summarizes key takeaways from the book Machine Learning for Factor Investing, which focuses on machine learning applications in factor investing and portfolio construction.
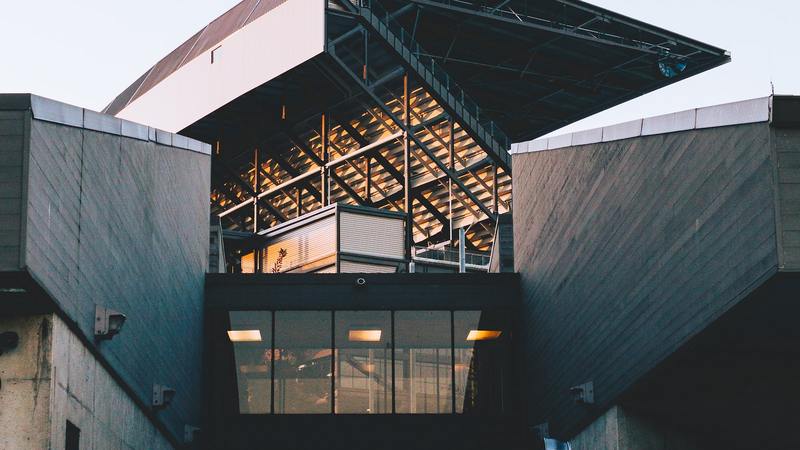
Machine learning helps discover new factors and relationships
One of the main benefits of machine learning is its ability to detect nonlinear relationships and interactions between company fundamentals and stock returns. For example, deep neural networks can be trained on company financials to forecast future fundamentals, which can then be used to select stocks in a factor investing strategy. So machine learning provides a data-driven way to discover new factors.
Machine learning models adapt better to changing markets
Markets are dynamic, so predictive patterns tend to change over time. Machine learning models like neural networks can be retrained dynamically on recent data. This allows the model to adapt to evolving market conditions. On the other hand, traditional linear factor models remain static over time.
Feature engineering is key to model performance
The data inputs into machine learning models significantly impact their performance. Extensive feature engineering with both price and fundamental data is essential. The book emphasizes how thoughtful input selection and preprocessing can be more important than the choice of machine learning algorithm.
Causality is difficult to establish in finance
Machine learning detects correlations and patterns in data, but discerning true causality is difficult. The ‘no free lunch’ theorem implies analysts should have an economic rationale behind models. Making reasonable assumptions about the response variable and features is critical.
Practical experience is indispensable
Despite sophisticated algorithms, practical experience with financial data is irreplaceable. Collecting data, coding backtests, tuning models, and debugging are indispensable steps. Hands-on practice iteratively improves intuition and results.
Machine learning is a valuable tool for factor investing, but needs to be grounded in financial theory and domain expertise. Thoughtful data preprocessing and feature engineering are just as crucial as model selection. Ultimately, practical experience is essential for applying machine learning successfully in finance.