Machine learning has made great progress in factor investing recently, benefiting from the developments of data availability, computing power and economic rationale. By detecting hidden patterns beyond asset pricing anomalies, machine learning models like lasso regression, random forest and neural networks can help construct more accurate factor exposure and improve portfolio performance. However, challenges still exist regarding noisy financial data, model interpretability, and out-of-sample efficacy.
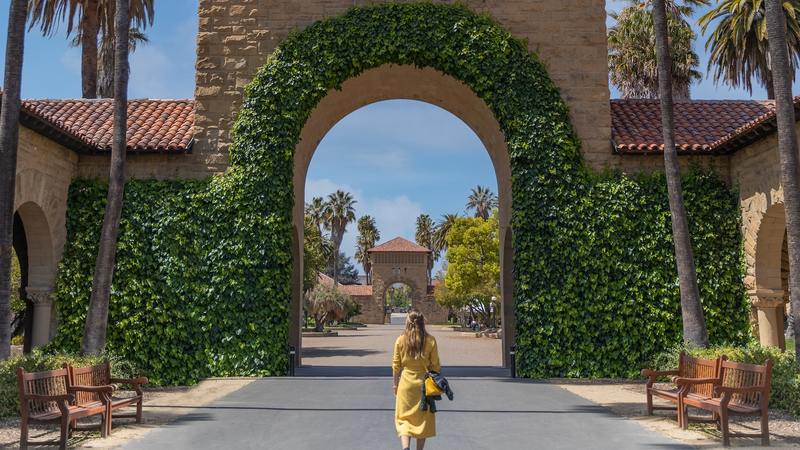
Machine learning models enable detecting hidden predictive patterns
The key premise of factor investing is that future returns depend on firm characteristics. These features have unknown and time-varying relationships with performance that machine learning is well suited to detect. By inputting a matrix of historical returns and hundreds of fundamentals, machine learning algorithms can discover nonlinear relationships and construct better factor portfolios.
Penalized regression produces sparse factor portfolios
Methods like lasso and elastic net regularization enable automatic variable selection and shrinkage, outputting a sparse set of stocks with key factor exposures. This drives the outperformance and differentiation of factor portfolios constructed using such techniques. The predictive patterns detected are also more robust to sample splitting.
Tree-based methods reveal factor relevance
Decision tree, random forest and gradient boosting machine models provide inherent feature importance ranking and visualization of factor relevance. The tree structure maps out return splits based on fundamentals like valuation, momentum and quality at different nodes. Such interpretability is useful for research even if predictive accuracy is similar.
Machine learning is a powerful toolkit for alpha generation, risk management and factor investing. But techniques require robustness to prevent overfitting and ensure efficacy out-of-sample. Noisy financial data remains a key challenge.