This article summarizes key information regarding investment strategies, return ratios, efficient portfolio construction and data analysis. By examining practical case studies, this essay elaborates upon the mathematical derivations and empirical results behind purportedly high Sharpe ratios. There are detailed discussions on mean-variance models, capital allocation techniques, margin trading, short selling and risk management. Multiple real-world examples help illustrate both proper validations and potential pitfalls when evaluating investment performance. The goal is to equip readers with a more nuanced understanding of investment theory and practice, so they can make more informed decisions.
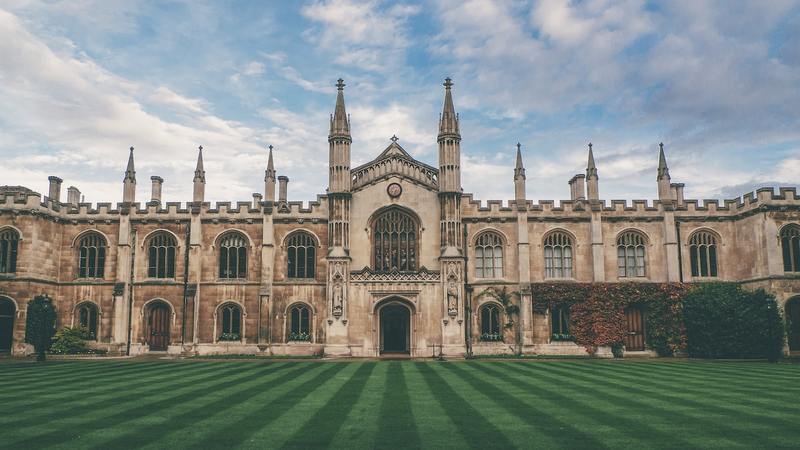
Using Nasdaq stocks, negatively correlated assets can produce double-digit Sharpe ratios
This section looks at the potential for high Sharpe ratios when including negatively correlated assets in a portfolio. Mathematical analysis and backtests reveal Sharpe ratios of over 10 are possible on paper by combining certain Nasdaq stocks over 1-2 year periods. However, some statistical issues like spurious correlations must be considered. As the lookback window expands, the minimum correlation and maximum Sharpe ratio decrease significantly.
Efficient frontier construction and optimization techniques
By minimizing portfolio variance and applying Lagrangian multipliers, the efficient frontier for a given set of assets can be constructed. Numerical methods and solvers facilitate optimization in Python and Excel. This section explains practical implementations, as well as the concepts behind capital allocation lines and tangent portfolios.
Leverage, shorting and risk management
Using margin, short selling, and value-at-risk constraints can substantially improve portfolio efficiency. Empirical results quantify potential reductions in risk versus reward. This section also covers risk decomposition and evaluation of ex-ante vs ex-post returns to avoid pitfalls.
Multi-asset analysis across indexes and geographies
Expanding the analysis to major global equity indexes reveals crucial considerations regarding data selection. Optimal capital allocation varies significantly depending on timeframe chosen. This section concludes with a discussion on the assumptions behind Markowitz portfolio theory and covariances.
In-depth coverage of mean-variance portfolio optimization provides a more nuanced look at investment strategy evaluation. While high returns are hypothetically possible, proper risk management and statistical significance testing is essential. This summarizes key mathematical derivations, implementation details, and empirical results using real-world data.