Investment research relies heavily on data analysis. The background samples used provide essential context for justifying methodology choices. This article examines data and model specifications from an economics research paper, offering insights into properly introducing materials/methods, providing specific details, relating approaches to other studies, and indicating problem areas. There are key principles for effectively presenting data sources, sample selection criteria, empirical specifications, and control variable definitions centering around the investment sample terminology.
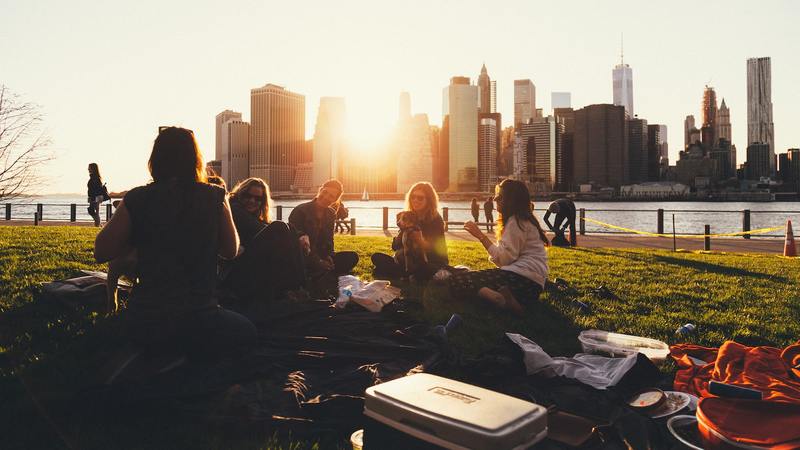
Justifying choices in investment sample methodology
The Giroud (2013) paper on proximity and investment sourced manufacturing plant data from three U.S. Census Bureau datasets. This enabled plant-level panel analysis over 29 years. The annual and longitudinal coverage of hundreds of thousands of plants was cited when describing the data. Explicit justification was provided for supplementing these datasets with headquarters location information from other Census Bureau data to facilitate distance calculations. The criteria for the final sample of 1.3 million plant-year observations was outlined, anchored around having at least two consecutive years of data for each plant. The paper acknowledged imperfections in headquarters data over time, but indicated appropriate care in handling this limitation to avoid bias.
Providing specifics on origins and uses of investment samples
The Giroud paper was precise in delineating the source of airline route data as DOT Form 41 filings that airlines operating U.S. flights must submit by law. It specified that these filings cover all domestic flights and gave examples of data fields like departure airports, flight durations, passengers, etc. This level of detail helps establish authority and enables reproducibility. Similarly, the introduction of each manufacturing dataset provided specifics on regularity of data collection, probability sampling approaches, typical numbers of establishments covered, mandatory reporting requirements, and particular variables captured. Identifying legal reporting mandates also aids reliability.
Investment sample methodology choices and legacy studies
Relating sampling and modeling decisions to existing literature helps frame investment analyses. The Giroud paper filtered out certain manufacturing plant observations following, as it cited, “common practice in the literature.” This defense of excluding imputed records and plants with zero/missing employment makes the sample more standardized. When discussing the selection of a 10-year window around treatment dates with flexibility in the interval length, the paper explicitly stated the robustness of results to window size as in prior studies. Such connections provide justification and context that reviewers and readers familiar with the domain literature will recognize.
Indicating investment sample limitations
Being upfront about imperfections in background samples can demonstrate thoughtful perspective in an investment analysis. The Giroud paper conceded that relying on the latest available HQ Auxiliary Establishment Survey for missing historical location data introduces noise. But it balanced this with arguments about the lack of bias due to Census year determinism and the conservative nature of still finding significant effects. The paper also noted that results are similar when including firms changing HQ location, heading off a specificity concern. Directly stating data aberrations, their analytical consequences, and sensitivity checks renders methodology more scientifically sound.
Investment analyses must carefully introduce data sources and define samples with exacting detail on origins, coverage, reporting mandates, variables, and continuity over time. Methodological choices should be explicitly justified based on accounting for key analytical issues and serving research objectives. Connections to legacy domain studies further ground approaches. Transparently indicating background sample limitations while arguing why findings remain valid enhances scientific credibility.