Investment analytics has become increasingly important in the financial industry. With massive amounts of market data, quantitative analysis like regression analysis, Monte Carlo simulation, and machine learning algorithms can help investors make better investment decisions. Analytics can help construct optimal portfolios, identify mispriced assets, develop profitable trading strategies, and manage risks. This article will illustrate how investment analytics powers various applications in investing.
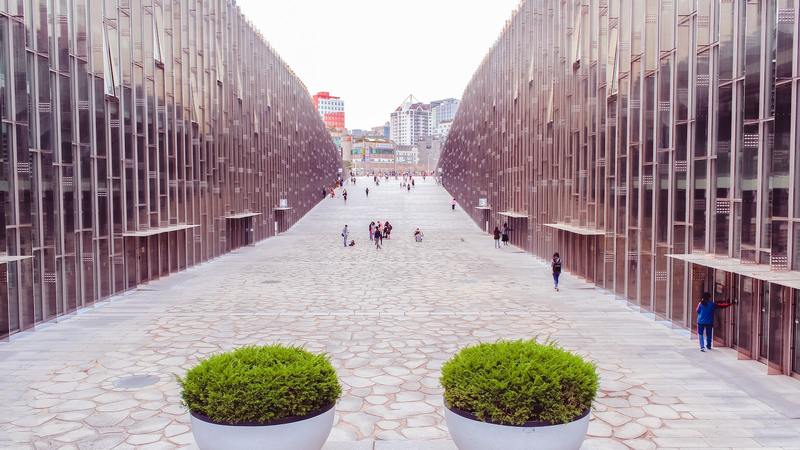
Regression models predict stock returns
Regression analysis is one of the most basic quantitative techniques used in investment analytics. By examining the relationship between stock returns and factors like market risk, firm size, valuation ratios, past returns etc, regression models can estimate the expected returns of stocks. The regression outputs like alpha and beta can help identify undervalued stocks to include in a portfolio. An investor can tilt their portfolio towards stocks with high expected returns based on the regression forecasts to try to beat the market.
Monte Carlo simulation optimizes portfolios
Monte Carlo simulation is an important analytical tool for portfolio optimization and risk management. By generating thousands of randomized market scenarios, a Monte Carlo simulation can model the range of possible portfolio outcomes. This allows an investor to construct the asset allocation that maximizes returns at every risk level. The simulation results can also be used to stress test a portfolio strategy and estimate maximum losses in a market crash. Monte Carlo methods provide probabilistic insights that are lacking in deterministic models.
Machine learning uncovers alpha opportunities
With computing power and big data, machine learning is transforming investment analytics. Algorithms can uncover complex non-linear patterns in massive datasets that humans cannot easily detect. Machine learning techniques like neural networks and deep learning can be used to make predictions, identify anomalies, and classify investment opportunities. For example, natural language processing can analyze news and social media sentiment data to generate trading signals. Cluster analysis can group stocks with similar characteristics as potential investments. Machine learning provides an edge for quants to generate alpha.
Quantitative techniques empower investment analytics across industries and strategies. Regression analysis, simulations, machine learning give investors unique insights from data to enhance returns. Analytics will continue growing in importance for finance.