With the rapid development of large language models like GPT-3 and GPT-4, there has been growing interest in how these AI models can be applied to investment analysis and decision making. GPT models have shown promising capabilities in extracting insights from huge amounts of textual data, generating investment ideas, quantifying market sentiment, and even directly managing portfolios. As costs of training and deploying GPT models decrease, financial institutions are accelerating research and adoption of these AI technologies. This article will analyze the current state and future trends of leveraging GPT in stock analysis, algorithmic trading, quant research, portfolio management, and other investment fields.
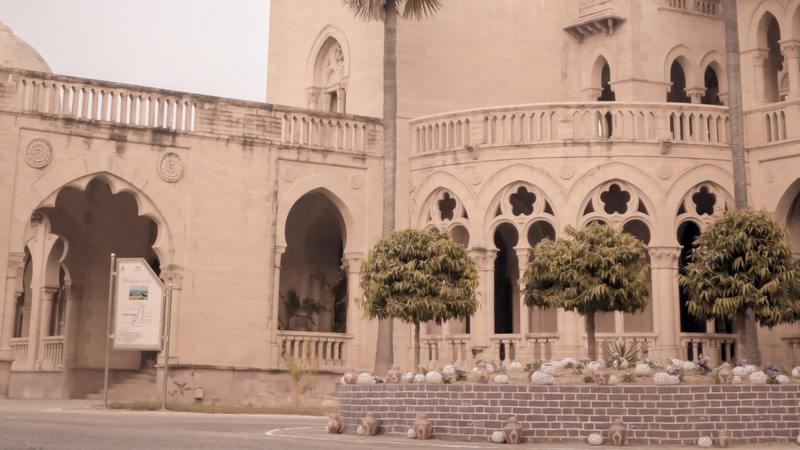
GPT drives more efficient information extraction and analysis for investments
One major advantage of GPT models is their superior natural language processing ability, which allows extracting key information and insights from various textual sources much more efficiently compared to humans reading through them. For example, GPT can read earning calls, financial reports, news articles, analyst reports across thousands of companies and summarize the most relevant points in minutes. This enables investors to absorb market data and events in a more timely manner. GPT also shows promising accuracy in predicting future earnings and stock prices based on past financial texts. With further training on finance domain data, GPT is expected to become an indispensable assistant for investment research and analysis.
GPT generates alpha-seeking investment ideas
GPT models can take a creative role in the investment process by generating novel alpha-seeking ideas. With ingestion of rich textual data across sectors, geographies and asset classes, GPT models can make investment connections that are non-obvious to humans restricted by biases and limited knowledge. For example, GPT may discover inter-industry relationships, macroeconomic leading indicators, or Geographic arbitrage opportunities that most analysts overlook. GPT idea generation provides a fertile starting point for human investors to further develop investable strategies. As training data expands to cover wider markets, GPT has the potential to become an AI powerhouse for systematic investing strategies.
GPT quantifies sentiment and risk factors for investment decisions
Sentiment analysis and risk quantification are crucial for investment decisions but prone to human bias and error. GPT models demonstrate increasing accuracy in digesting volumes of textual content to quantify market psychology, corporate tone, geo-political risks and ESG factors. For instance, GPT sentiment analysis on management tone during earnings calls has shown high correlation with future stock price movements. Quantifying subjective factors allows investors to enhance risk-return modeling. With further development, GPT could become integral to quant funds for sentiment and risk analytics across enormous datasets.
GPT enables lower-cost active portfolio management
As GPT models advance, they are expected to have increasing autonomy in portfolio management traditionally done by human teams. GPT can actively adjust portfolio asset allocation and security selection based on analysis of latest market data. Key benefits compared to traditional active management include: vastly larger analysis scope; freedom from human biases; 24/7 working capability; and lower labor costs. Although unlikely to fully replace human oversight and decision making in the near future, GPT is poised to democratize sophisticated active portfolio management. Investors of all sizes can benefit from GPT-powered robo-advisors and hedge fund alternatives.
In summary, GPT models are driving transformative change across the investment landscape by enabling more efficient information extraction, novel idea generation, sentiment and risk analytics, lower-cost active management, and much more. As costs keep decreasing, capabilities improve, and applications expand, GPT is set to become an increasingly integral component of the investor toolkit.