With the development of machine learning and artificial intelligence, using model ensembles has become an important technique in quantitative investment research. Ensemble methods combine multiple models to improve predictive performance compared to a single model. Recent studies have shown that model ensembles can effectively improve investment returns and risk management. This article will examine how integrating multiple machine learning models can better capture market information and generate robust investment signals. Specifically, we will analyze cases of combining reinforcement learning models in algorithmic trading, as well as integrating linear and non-linear models in factor investing.
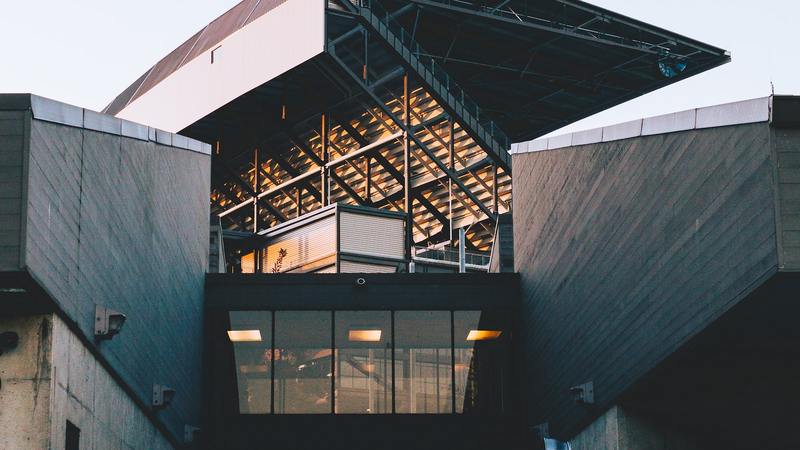
Ensemble reinforcment learning models enhance trade execution
In algorithmic trading, reinforcement learning (RL) models have been increasingly used for making trading decisions. However, a single RL model often cannot fully capture the complexity of financial markets. Researchers have found ensemble approaches that integrate different RL models can better adapt to changing market regimes. For example, Zhang et al. (2022) combined A2C and PPO models for trading stock index futures. The two models were backtested separately first. During live trading, their performances were evaluated in rolling windows to select the best model for the next time period. This ensemble approach dynamically chose the optimal model conditioned on the market state, achieving higher overall returns than individual models.
Combining linear and non-linear models extracts hidden factors
In factor investing, standard linear factor models have theoretical limitations in capturing non-linear relationships in stock returns. Recent studies have proposed using machine learning models to uncover non-linear factors from residuals unexplained by linear models. For example, Bonne et al. (2021) used random forests, neural networks and other ML models to fit the specific returns from the Fama-French 5-factor model. The exposures to the resultant ML factors were then combined into an ensemble, which showed significant explanatory power for the cross-section of returns. Such integration of linear and non-linear models provides a promising approach to extract previously hidden sources of systematic risk.
Applying model ensembles by integrating different types of investment models is an effective technique to improve performance. Combining reinforcement learning agents adapts trading systems to changing markets. Integrating linear and machine learning models better explains cross-sectional stock returns.