Directional change (DC) analysis is an emerging method in quantitative investment research. By setting appropriate thresholds, DC analysis can effectively capture price fluctuations caused by events and make predictions about future price trends. This provides useful incremental signals for stock selection and trading strategies. In this article, we will walk through the construction of DC models and improvements, as well as empirical evidence on the application of DC analysis in the Chinese stock market. There are wide applications of DC methods in quant investment strategies by characterizing meaningful price moves and predicting post-event stock returns.
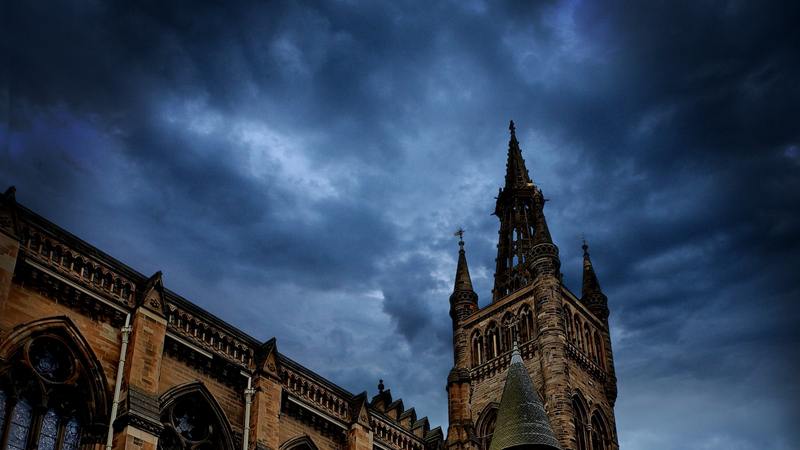
DC analysis constructs models by summarizing price extremes
The key idea of DC analysis is to characterize all price extremes based on pre-set thresholds, filtering out transitional fluctuations in between. This works well in describing complex price movements while reducing data noise. Specifically, a DC event is identified when the price change between two extremes exceeds a threshold theta. The price move from one extreme to the next DC confirmation point is defined as a DC event. DC methods aim to capture such events that significantly impact price, like convertible bond issuance and directors’ share purchase.
DC strategies generate excess returns in empirical studies
Empirical studies demonstrate that DC-based trading strategies can harvest risk-adjusted excess returns in the Chinese stock market when proper estimation and investing windows are set. One study shows that the optimal theta is time-varying and sensitive to short estimation windows. The strategy performs the worst with 1-year estimation and investing windows. As the estimation window gets longer, the optimal theta converges and the strategy return improves. Overall, medium-to-long-term DC strategies with 3-5 year estimation windows are more suitable in China. However, adjustments may be needed for short-term DC strategies.
Dynamic thresholds further improve model accuracy
The traditional DC method uses a fixed threshold, which fails to adapt to varying event magnitudes. Recent studies propose a dynamic threshold approach that computes the threshold based on prior day price moves and next day open. Backtests show the dynamic threshold DC model perfectly matches all events confirmed by news, while fixed threshold models have high error rates in detecting events, proving superior accuracy.
DC analysis provides useful signals for stock investments
To conclude, DC analysis is an event-driven method that models market price movements by summarizing extremes. It serves as an incremental alpha signal on top of existing factors. With proper threshold setting and window lengths, DC strategies can generate significant risk-adjusted excess returns in stock investments. But in practice, dynamic thresholds are needed to accurately detect events in real time. The applications of DC analysis in quant investment deserve further research.
Directional change (DC) analysis captures price fluctuations caused by major events and makes predictions about post-event return trends. DC strategies can generate excess returns in stock investments when proper thresholds and estimation/investing windows are set. Dynamic threshold DC models outperform fixed threshold models in detection accuracy. DC analysis provides useful incremental signals for stock selection and trading strategies.