Data science is transforming the field of investing and reshaping how investment decisions are made. With vast amounts of market data available, data science techniques like machine learning and predictive analytics can uncover hidden insights and enable more informed investing. This article will explore how data science is being applied in finance and provide examples of data science investing strategies. An overview of key data science methods for investing will be provided along with real-world case studies. The vital role data science now plays in investment research, algorithmic trading, risk management and portfolio optimization will be discussed. By unleashing the power of big data, data science is leading a quantitative revolution in the investment world.
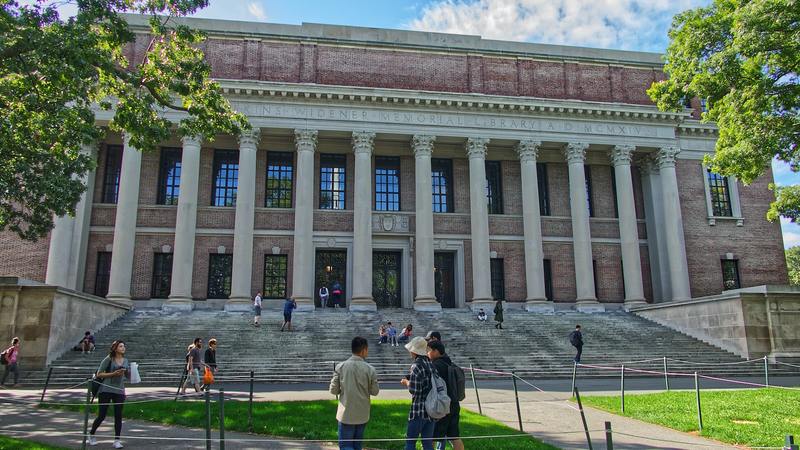
Machine learning algorithms for predictive analytics
One of the most common applications of data science in investing is using machine learning algorithms to make market predictions. By analyzing large datasets of historical asset prices, news events, earnings reports and other factors, machine learning models can uncover predictive patterns. These models can then forecast future price movements, identify mispriced assets and generate profitable trading signals. For example, neural networks are adept at finding complex nonlinear relationships in financial data that may be missed by traditional regression models. Deep learning networks with many layers can detect subtle market patterns that lead to accurate predictions of returns.
Data mining for actionable insights
Data mining techniques are invaluable for discovering actionable insights from the vast troves of market and consumer data available today. Methods like clustering analysis can segment the market and find distinct groups of assets, securities or even investors that behave similarly. Association rule learning can uncover interesting correlations in the data that may point to profitable trading ideas. By thoroughly data mining many data sources using different techniques, investors can gain a informational edge over the broader market.
Sentiment analysis of news and social media
Analyzing market sentiment based on news headlines, financial reports and social media chatter is now an essential part of any data science investing strategy. Using natural language processing and text analysis, the tone and emotions within textual data can be measured. A negative sentiment score may signal trouble ahead for a company while a spike in positive sentiment may indicate rising investor enthusiasm. Hedge funds commonly employ advanced sentiment analysis to generate timely trading alerts that capitalize on shifting market sentiment.
Algorithmic trading strategies
Data science is behind the rise of algorithmic trading and automated trading systems. Complex machine learning models are developed to monitor markets, identify trading opportunities and execute trades faster than any human. Quantitative algo trading strategies powered by data science are employed across Wall Street to trade stocks, options, futures and currencies. These strategies are constantly learning and improving by crunching vast amounts of market data. And emerging deep learning techniques are being applied to find even more subtle patterns and profitable strategies.
Portfolio optimization and risk analytics
Modern portfolio theory is being augmented using data science techniques for portfolio optimization. By applying machine learning to risk analytics, portfolios can be structured to maximize returns at every level of risk. Big data and clustering algorithms can identify assets with similar risk attributes to construct well-diversified portfolios. Stocks can be ranked by machine learning models to build portfolios resistant to market declines. With data science, investors can continually optimize their portfolios as the market changes over time.
Data science is transforming investing by enabling powerful quantitative analysis of vast amounts of market and consumer data. Techniques like machine learning, data mining and sentiment analysis are generating actionable insights for investment research and trading. And data science now underpins algorithmic trading, portfolio optimization and risk management across the finance field. The data science investing revolution has only just begun as new methods and data types continue to emerge.