Black box investment strategies, also known as quant strategies, refer to algorithmic trading systems that make investment decisions based on quantitative models and algorithms. They are considered ‘black boxes’ because the logic behind the models is not transparent to outsiders. These strategies have become increasingly popular in recent years due to advances in computing power and the availability of big data. However, they also come with certain limitations and biases that need to be understood.
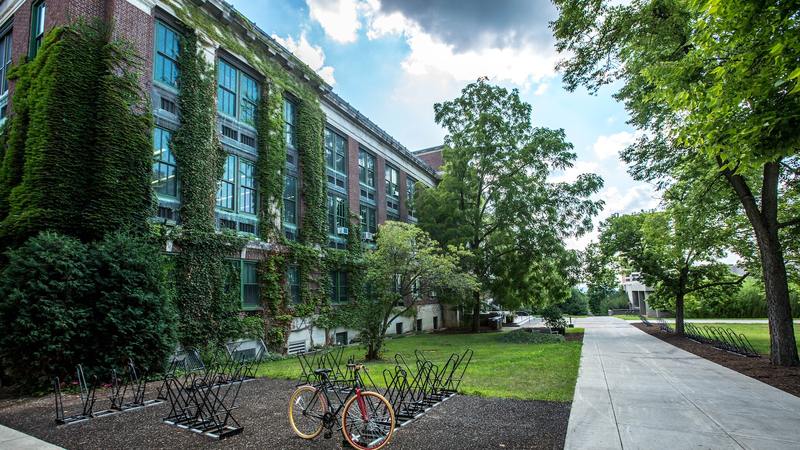
Different components of a black box trading system
A quantitative trading system typically consists of three key components – an alpha model, a risk model, and a transaction cost model. The alpha model aims to generate profitable signals, while the risk model controls for different types of risk exposures. The transaction cost model estimates trading costs like commissions and slippage. These components then feed into a portfolio construction model that determines position sizes. Finally, the orders are executed through a separate execution model.
Common strategies used in black box models
Some common algorithmic strategies used in black boxes include trend following, mean reversion, arbitrage, machine learning models and more. Trend following aims to identify and profit from momentum in asset prices over different time frames. Mean reversion looks to exploit distortions from fair asset values. Arbitrage seeks to capitalize on differential pricing of similar assets. Machine learning models can also detect complex patterns from large datasets.
Biases and limitations to be aware of
While black box models offer many advantages, they also come with certain biases like optimization bias, survivorship bias and look-ahead bias. Optimization bias stems from excessive curve-fitting on historical data. Survivorship bias arises when unsuccessful strategies are excluded from the backtest. Look-ahead bias happens when future information is inadvertently used. Black boxes may also underperform in regime shifts and have opacity risks from model uncertainty.
Sourcing trading ideas for algorithmic models
Some ways to source profitable trading ideas include academic papers, blogs, forums as well as independent research requiring skills in market microstructure, machine learning and more. It’s also important to properly evaluate strategies on metrics like risk-adjusted returns, volatility, maximum drawdowns before implementation.
Black box quantitative strategies make up a major part of algorithmic and high-frequency trading today. While offering advantages like backtesting capability, they also come with biases and limitations around optimization, survivorship and look-ahead bias. Sourcing profitable ideas requires both market knowledge as well as programming and data skills.