Black box investment strategies refer to quantitative, model-based approaches that make investment decisions automatically based on mathematical models and algorithms. As the models operate like a ‘black box’ without transparency of the underlying logic, they are called black box strategies. Such data-driven strategies have become popular in recent years due to the proliferation of computing power and digital data. Though lacking interpretability, black box models can efficiently process huge amounts of data to uncover complex patterns beyond human perception. This article provides an analysis of various aspects of black box investment strategies.
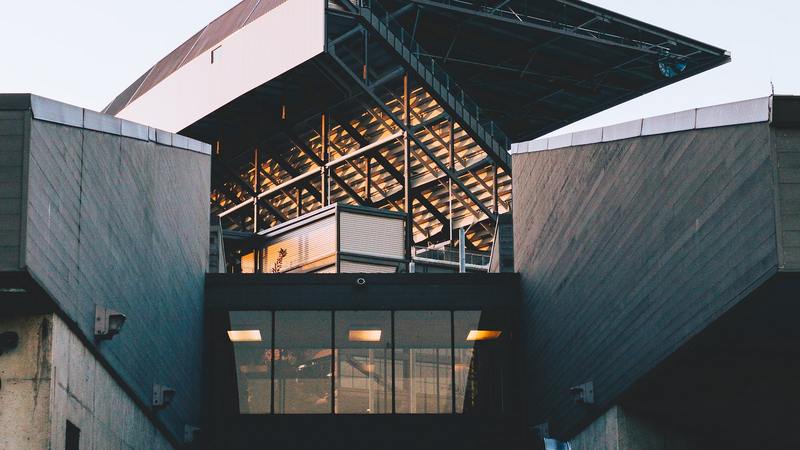
Definition and strengths of black box investment strategies
Black box investment strategies utilize mathematical and statistical models to make investment decisions, without the need for human judgement or emotions. The models operate based on historical data like price movements, volatility indicators and trading volumes. Some of the major strengths of such model-based quantitative approaches include: 1) Emotion-free decisions grounded in hard data, 2) Ability to process huge datasets efficiently to uncover hidden predictive signals, 3) Rapid and consistent execution of trades as per model signals, and 4) Easy backtesting on historical data and optimization of the systems.
Types of models used in black box trading systems
There is a wide variety of quantitative models that can be used to develop black box trading systems, including: 1) Statistical arbitrage – Using cointegration and correlation of instruments to profit from deviations from historical norms, 2) Machine learning models like neural networks that can uncover complex nonlinear patterns from data, 3) Reinforcement learning agents that optimize their trading policy based on environment rewards and penalties, 4) Evolutionary algorithms that can create and evolve diverse trading rules based on principles of genetics and natural selection.
Risk management considerations for black box strategies
While black box models have several advantages, some key aspects must be considered from a risk perspective when using such systems: 1) Overfitting – Models tuned excessively to historical data may fail on out-of-sample data. Rigorous validation is essential. 2) Unexpected market regime changes – Models relying purely on quantitative data may not perform well when fundamental market dynamics shift. Human oversight is key. 3) Transparency and auditability – Lack of model interpretability necessitates compensating governance and controls. 4) Cybersecurity – Attacks targeting confidential trading algorithms and data can have catastrophic outcomes.
Future opportunities and challenges
Going forward, increasing availability of alternative datasets from sources like satellites, Internet-of-Things sensors and online platforms provide new signals to enhance black box models. Advances in computational techniques like cloud computing and graphics processors enable faster development and deployment of complex strategies. However, concerns around fairness, transparency and job losses due to automation present important challenges for the industry that necessitate collaborative solutions.
In summary, black box investment strategies hold great promise to augment alpha generation and risk management, but also pose unique technological and ethical problems that the finance industry needs to tackle. Thoughtful design and oversight of such data-centric systems can help realize their benefits while mitigating the downside risks.