With the growth of factor investing in recent years, fixed income portfolio managers have started utilizing multi-factor models to better control risk exposures and potentially generate excess returns. Factors like value, momentum, carry, liquidity etc. have proven effective in equity markets and are now being applied in global bond markets as well. By combining macro factors that track risk premiums with style factors that target mispricings, investors can build robust fixed income portfolios. This article explores some of the best practices for fixed income factor investing.
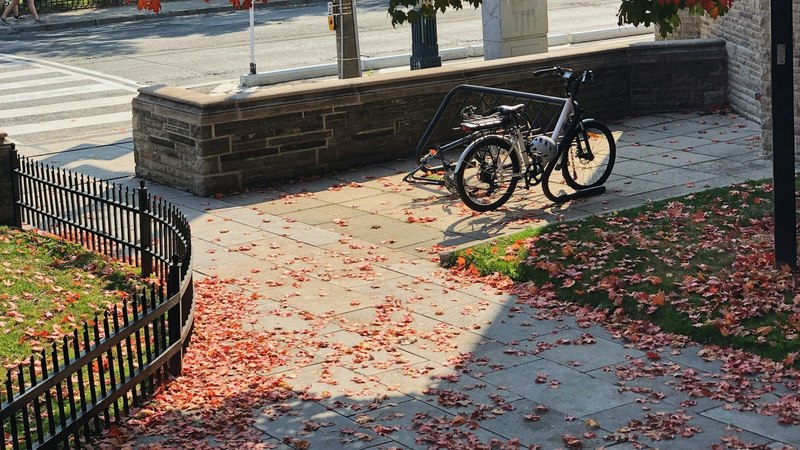
Utilizing macro factors like value and momentum in addition to duration can enhance risk-adjusted returns
While matching the duration of a benchmark is important for controlling interest rate risk, adding exposures to bond market value and momentum factors has been shown to improve Sharpe ratios historically. Value strategies favor higher yielding, cheaper bonds based on yield spreads or other valuation measures. Momentum signals can help position portfolios for yield curve shifts and ride price trends in credit markets.
Careful bond picking based on relative value and events can further augment performance
On top of strategic macro factor allocations, fixed income managers can pursue excess returns through security selection. Comparing valuations across on-the-run/off-the-run Treasury bonds, sectors, credits and yield curve maturities can uncover mispricings. Around corporate events like earnings or M&A, debt instruments often react inefficiently allowing nimble managers to capitalize.
Blending both active and passive exposures allows for better risk control
Rather than a purely active or passive approach, combining indexed exposures that track benchmark factors with an actively managed overlay portfolio that seeks mispriced securities may optimize performance. The indexed component controls systematic risks cost efficiently while the active sleeve provides potential alpha. Managers can shift the weighting between active and passive to flexibly adapt to changing market conditions.
Regular rebalancing and risk model updating maintains stability amidst market shocks
As quantitative signals and price relationships change, fixed income portfolios need periodic rebalancing and optimization to stay consistent with return targets and risk limits. Updating multi-factor risk models using machine learning techniques helps keep pace with evolving correlations and volatility clusters seen during crisis events.
By augmenting mainstream index-tracking approaches with multi-factor models and security selection skill, fixed income managers can build portfolios positioned to outperform benchmarks over the long run on a risk-adjusted basis.