Artificial intelligence has brought transformative changes to the investment research field. With massive data processing capabilities, AI can extract insights and generate alpha that were not possible before. This article will examine how AI enhances investment research through alternative data analytics, predictive modeling, sentiment analysis, risk management, and portfolio optimization. Proper application of AI provides institutions and individuals a competitive edge in the market.
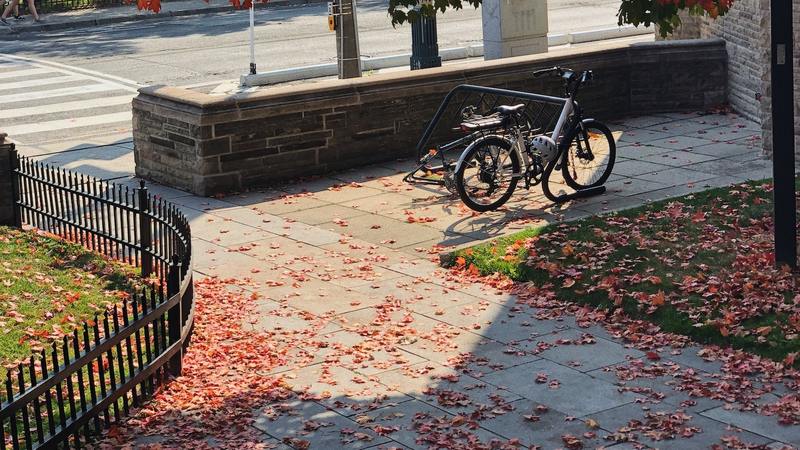
AI alternative data analysis expands information for investment research
Traditional investment research relies heavily on conventional data sources like financial statements, analyst reports, and macroeconomic indicators. AI opens up previously unavailable alternative data for gaining investment insights. This includes satellite imagery, credit card transactions, web traffic, social media posts, and more. Powerful machine learning algorithms can process these massive unstructured datasets to identify patterns not captured by conventional analytics. Hedge funds and asset managers are aggressively adopting AI-powered alt data analysis to supplement traditional research.
AI predictive modeling improves investment forecasting accuracy
Predictive modeling is another key application of AI in investment research. Algorithmic models can analyze cross sections of financial data to detect predictive signals. With neural networks, the models continuously self-improve their forecasting accuracy. Quantitative investors build stock return, volatility, cash flow, earnings, and other predictive models using AI to generate profitable trading strategies. The machine learning predictions augment or even replace human projections that rely on subjective assumptions.
AI sentiment analysis provides value investor intelligence
Analyzing qualitative data like financial news, earnings call transcripts, or social media feeds is critical for an insightful investment research process. But the amount of textual content overwhelms human capacity. AI sentiment analysis automates this process by using NLP algorithms to parse text data and quantify narrative sentiment. The machine learning models can track management sentiment, investor sentiment, consumer sentiment, and expert opinions on a vast scale. This allows investors to supplement valuations with a data-driven assessment of intangible variables.
AI risk management improves portfolio resilience
Portfolio risk management is another key area where AI is transforming investment research. By processing massive amounts of historical data, machine learning algorithms can backtest portfolio performance over decades of market events. This enables stress testing under extreme scenarios and improves capital preservation. AI-based VaR models provide more accurate risk measurement compared to traditional models. Algorithmic optimization also allows constructing robust portfolios resilient to economic shocks and volatility.
AI portfolio optimization uncovers novel opportunities
The massive processing power of AI allows asset managers to continuously optimize their portfolios in line with changing market dynamics. Machine learning algorithms can analyze millions of securities for optimal weight allocation that maximizes returns at intended risk levels. This allows dynamically improving portfolio construction as new information becomes available. AI helps uncover non-intuitive asset combinations neglected by traditional fund management approaches. It enables quantitative investors to capitalize on novel alpha opportunities through systematic portfolio optimization.
AI is transforming investment research by enabling alternative data analytics, predictive modeling, sentiment analysis, risk management, and portfolio optimization. Proper application of machine learning provides institutions and individuals a competitive edge with data-driven insights.