In today’s volatile financial markets, having a static investing strategy can be risky. An adaptive investing strategy dynamically adjusts your portfolio holdings and allocations based on changing market conditions. This allows investors to mitigate risks and capitalize on emerging opportunities. By incorporating data analytics and machine learning, adaptive investing aims to automate the portfolio adjustment process. We will explore popular frameworks like tactical asset allocation and risk parity. Understanding how to implement an adaptive strategy can help investors navigate uncertainty and enhance long-term returns.
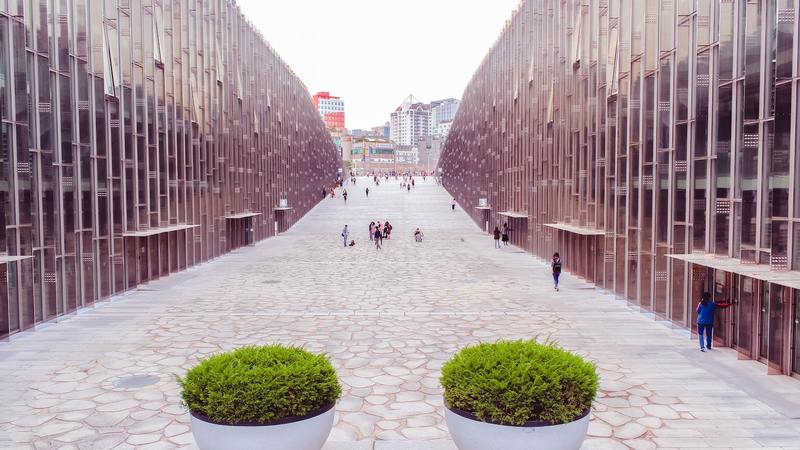
Tactical asset allocation dynamically shifts allocations
Tactical asset allocation (TAA) is a framework for dynamically adjusting portfolio allocations across asset classes based on their expected risks and returns. For example, when stock market valuations appear high, a TAA strategy may reduce equity exposure and rotate into fixed income or cash. Various signals like valuation ratios, economic indicators, and momentum can inform TAA decisions. By tilting away from overpriced assets, investors hope to enhance risk-adjusted returns over a market cycle. Some challenges are frequently trading generates transaction costs and signals may be unreliable. Overall, combining TAA with strategic asset allocation provides a balance of dynamism and discipline.
Risk parity aims to equalize risk contributions
Risk parity investing equalizes the amount of total portfolio risk that each asset class contributes. This means higher volatility assets like stocks get smaller allocations than bonds. For example, a basic 60/40 portfolio concentrates over 90% of risk in equities. Risk parity would drastically cut equity exposure to reduce concentration risk. This approach differs from allocating by capital, as with classic 60/40. Some benefits are diversification and resilience in crises when stocks fall together. Challenges are high leverage required to achieve parity and poor performance when bonds decline. Intelligently leveraging diversification can enhance risk-adjusted returns.
Machine learning models create adaptive portfolios
Recent advances in machine learning and big data allow investors to create highly adaptive quantitative strategies. These model-driven approaches analyze a breadth of data to inform investment decisions and portfolio adjustments. For example, neural networks can monitor various risk factors, economic indicators, and prices for patterns. The models then automatically rebalance holdings, tilt exposures, or hedge risks based on insights. With massive datasets and advanced algorithms, machines can potentially find complex relationships and capitalize on evolving opportunities. Challenges are model risk, overfitting, and intelligibility.
Rather than sticking to static asset allocations, adaptive investing dynamically adjusts portfolios to changing market environments using frameworks like TAA, risk parity, and machine learning models. This provides the benefits of tactical positioning and risk management. However, these complex and frequent adjustments also pose modeling, transaction cost, and misalignment risks to monitor.