In the field of investment banking, accurately predicting stock prices is crucial yet challenging. When examining models like ARIMA, RNN/LSTM, and machine learning, they all have certain limitations in capturing the complex relationships in financial markets that impact share prices day to day. Factors like investor sentiment, macro events, black swan risks all contribute to volatility. This article analyzes the merits and downsides of various predictive modeling techniques, and argues that while helpful, currently no approach can reliably and precisely forecast near-term stock fluctuations.
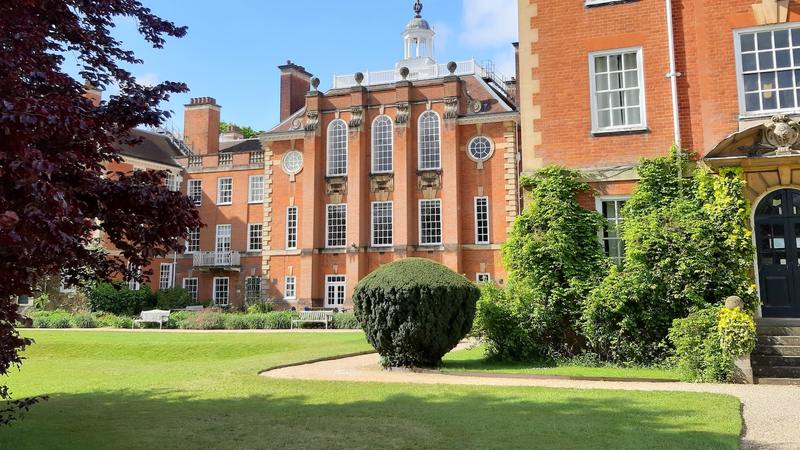
ARIMA effective for short-term stock prediction but has limitations
The ARIMA model can perform well for short-term stock price forecasting by comparing predicted values to actual. However, it only considers the time series itself without external factors. Thus ARIMA performs poorly on volatile series. Overall, linear ARIMA has limits capturing non-linear relationships in complex financial systems impacting stocks.
LSTM models show promise for time series but also limitations
LSTM models using deep recurrent neural networks seem promising for overcoming limitations of ARIMA. The “iterative” algorithm allows deeper learning from longer time series. However LSTM RNN models still have difficulty accounting for unseen data, tail risks, and rapidly evolving market conditions.
Accurately predicting today’s stock prices remains extremely difficult
Despite advances in predictive analytics, reliably forecasting day to day share price movements remains extremely difficult. No model can fully account for investor emotions, breaking news, geopolitics, acts of nature etc. Caution should be taken with any services claiming to offer precise short term stock predictions.
Accurately predicting investment banking stock prices on a day to day basis is extremely challenging and no current model is reliable enough to replace human oversight and discretion. A prudent mix of quantitative analytics and qualitative human judgement is advised.