Pythagoras fuzzy set theory and related decision making methods have shown great application value in the field of investment analysis and decision making. Compared with traditional methods, Pythagoras fuzzy set theory can better handle uncertainty and incomplete information in the investment environment. The key advantage lies in its ability to simultaneously consider membership degree, non-membership degree and hesitancy degree, thus providing a more comprehensive description of the fuzziness of investment objects or criteria. This article summarizes the basics of Pythagoras fuzzy sets, and introduces two representative case studies on how Pythagoras fuzzy decision making approaches are applied in venture capital investment selection and stock investment portfolio optimization, demonstrating the superiority and effectiveness of this emerging methodology in solving complex investment decision problems under uncertainty.
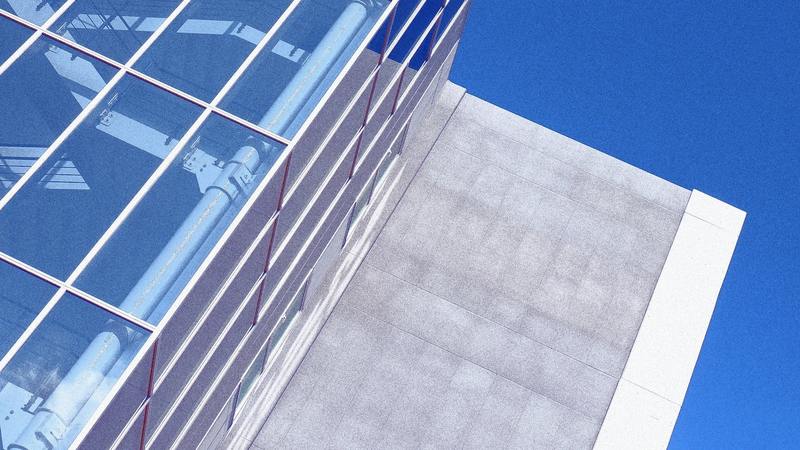
Key concepts and properties of Pythagoras fuzzy sets for investment modeling
The Pythagoras fuzzy set, proposed by Yager and Abbasov, is an extension of the intuitionistic fuzzy set. It introduces an additional parameter called “hesitancy degree” to depict the uncertainty and vagueness of objects. This trio of membership degree, non-membership degree and hesitancy degree must satisfy the condition that their square sum is not larger than 1. By simultaneously considering hesitation and fuzziness, Pythagoras fuzzy set provides a more comprehensive description of fuzziness than traditional fuzzy sets.
For investment analysis problems with complex criteria or incomplete information, Pythagoras fuzzy set theory has apparent advantages over existing methods:
1) The extra hesitancy degree enriches the representation model to better capture uncertainty;
2) Knowledge measure can quantify the information content contained in the Pythagoras fuzzy number. It lays the foundation for information aggregation in group decision making;
3) The monotonicity and boundedness of knowledge measure facilitate the normalization process in decision matrix construction.
Therefore, Pythagoras fuzzy set theory provides a useful mathematical framework to handle the innate complexity and ambiguity of investment objects and criteria.
Case study 1: Pythagoras fuzzy AHP for venture capital project selection
The first case analyzes how a venture capital firm leverages Pythagoras fuzzy set theory to evaluate and select from five bike-sharing startups to invest in, including Mobike, Ofo, Hellobike, Youbike and Yonganhang.
The evaluation process follows the steps below:
Step 1. Construct a hesitant Pythagoras fuzzy decision matrix based on scores from three experts in terms of four criteria – number of users, management capability, return on investment and public reputation. Normalize the matrix.
Step 2. Derive expert weighting vector and aggregate individual evaluations into a group decision matrix.
Step 3. Construct equivalent knowledge measure matrix. Determine criteria weight vector by maximizing the overall information content contained in the alternatives.
Step 4. Calculate the weighted aggregated Pythagoras fuzzy values of alternatives, and derive the ranking according to their knowledge measures. Select the one with highest overall score.
The result shows Hellobike as the optimal choice, followed by Mobike and Youbike. The whole process demonstrates the flexibility of Pythagoras fuzzy set in tackling uncertainty and incomplete information during project evaluation. The additional hesitancy degree also enables a more nuanced comparison between finely-grained differences of the alternatives.
Case study 2: Pythagoras portfolio optimization model for stock investment
The second case applies Pythagoras fuzzy theory to establish an optimistic and pessimistic fuzzy portfolio optimization model, aiming to maximize return and minimize risk for stock investment.
Compared to classical Markowitz mean-variance model, this Pythagoras model has following distinctions:
1) Use fuzzy numbers to describe the uncertainty of expected return and risk;
2) Maximize the satisfaction degree from both upside and downside to achieve tradeoff between profit and loss;
3) Derive the fuzzy efficient frontier with embedded uncertainty and flexibility.
Empirical test shows the proposed model provides a larger feasible region and gives more value-at-risk efficient solutions than classic methods. It offers investors better understanding of return-risk tradeoffs by translating uncertainty into intuitive membership satisfaction degrees.
To conclude, Pythagoras fuzzy set theory and its decision making approaches have demonstrated great effectiveness and superiority in handling complex investment decision problems with uncertainty and vagueness. The two case studies reveal promising application value across different fields including venture capital project selection and quantitative stock portfolio construction. More application areas can be explored and researched to facilitate technology transformation.
In summary, Pythagoras fuzzy set theory provides useful tools to model uncertainty and complexity inherent in the investment field. With the help of additional hesitancy degree and information aggregation approach, Pythagoras fuzzy decision making methods can effectively solve investment problems with incomplete information and inaccurate assessments. The case studies on venture capital and stock investment have validated the advantages and versatility of this emerging methodology.