Constructing an optimal investment portfolio is key to maximizing returns while minimizing risks. By analyzing historical returns, risks, correlations of various asset classes, investors can identify an efficient frontier of optimal portfolios that provide the highest expected returns per unit of risk. Various analytical techniques like the Capital Asset Pricing Model, Sharpe ratio analysis, Monte Carlo simulation etc. can help determine the asset allocation that aligns best with an investor’s risk appetite and return objectives. This article provides a step-by-step walkthrough of portfolio analysis techniques, illustrated using real-world data and examples. Proper portfolio analysis enables investors to make data-driven decisions to optimize their investment portfolio.
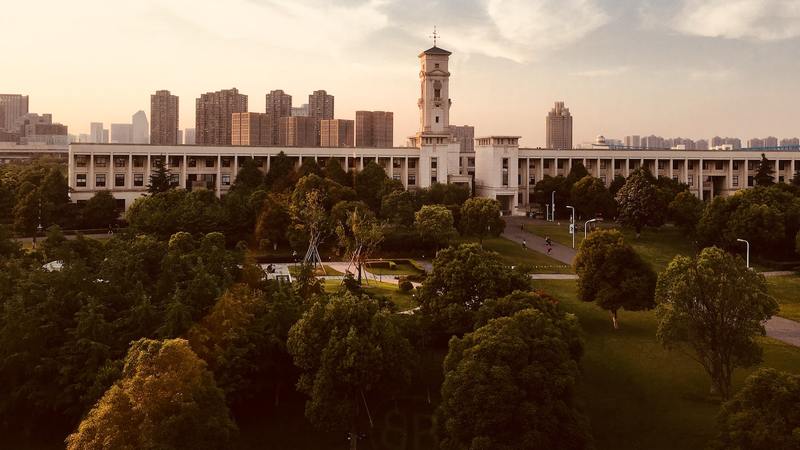
Use historical data to estimate asset class returns, risks and correlations
The starting point of portfolio analysis is gathering historical return data on various candidate asset classes for inclusion in the portfolio, such as stocks, bonds, real estate, commodities etc. By analyzing long-term return patterns, an investor can estimate the expected return and risk parameters like variance, standard deviation for each asset. Furthermore, computing correlations between asset class returns over time provides insights into diversification potential.
Apply mean-variance optimization to identify efficient frontier
The efficient frontier represents portfolios that offer the highest expected return for a given level of risk. By optimizing weighted asset class allocations to maximize return while constraining risk (variance), investors can systematically identify portfolios along the efficient frontier. This mean-variance optimization process can be implemented in Excel or using dedicated portfolio analysis tools.
Use CAPM to estimate required returns for assets
The Capital Asset Pricing Model provides a framework for estimating the required return for an asset based on its systematic risk relative to the market portfolio. By comparing an asset’s CAPM-implied required return to its historical return, investors can identify assets that may be undervalued or overvalued.
Analyze portfolios using Sharpe ratio and other metrics
Sharpe ratio evaluates return per unit of risk for a portfolio, enabling comparison of portfolios on a risk-adjusted return basis. Other useful metrics are alpha, beta, R-squared, tracking error. These portfolio analysis techniques help assess performance relative to benchmarks and suitability for an investor’s objectives.
Stress test portfolios using Monte Carlo simulation
Monte Carlo simulation models portfolio performance across thousands of simulated market scenarios, providing a statistical distribution of potential outcomes. Stress testing portfolios using Monte Carlo analysis reveals how well the portfolio allocation withstands tail risks like market crashes, supplementing traditional mean-variance techniques.
In summary, portfolio analysis leverages historical data, financial models and optimization techniques to construct portfolios with the highest risk-adjusted returns. A data-driven portfolio analysis process allows investors to backtest and stress test candidate portfolios before implementation. For long-term investing success, having a robust investment portfolio analysis framework is indispensable.